Staying ahead of the curve: How Generative AI is impacting Fintech
- Julio Gomez
- Mar 15, 2023
- 10 min read
Updated: Dec 18, 2023
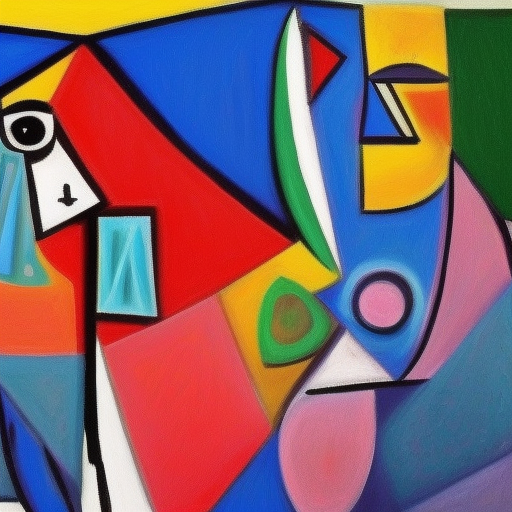
Generative AI has become critical in Fintech. It can improve customer experience, automate processes, and provide better insights (among other myriad of applications). Becoming AI-first will be a matter of survival for Fintech companies, and in doing so remain competitive in an industry that is well known for been rapidly evolving. In this article, we discuss how Fintechs are leveraging AI to gain a competitive advantage in the financial services industry.
AI technologies have improved exponentially in the last few years, and have revolutionised several industries. AI-powered machines can personalise recommendations for digital content, design fashion lines for retailers, and even outperform experienced doctors in cancer detection.
Generative AI is impacting Fintech
AI is rapidly becoming a mainstream technology, and the financial services sector has clearly been an earlier adopter. According to a 2020' survey by EY, 85% of respondents were already utilising some form of AI, with Fintech companies slightly ahead of traditional financial institutions in terms of adoption. When considering company size, Fintechs also allocate a higher percentage of their R&D budgets to AI.
According to the same survey, investment managers are at the forefront of AI adoption within financial services, primarily using the technology to generate new revenue streams. Payment providers follow closely behind, implementing AI mainly for process re-engineering and automation. The most prevalent application of AI across firms is however in risk management, used by 56% of companies, followed by revenue generation at 52%. Within two years, 95% of firms anticipate utilising AI to create new revenue opportunities.
Specific AI use cases include AI-powered data analytics (adopted by 43% of firms), fraud/anomaly detection and surveillance (42%), and AI-enhanced customer communication channels (36%). Fintechs tend to focus on leveraging AI to develop new products and services, while traditional financial institutions primarily use it to enhance existing offerings.
A trend towards mass AI adoption is emerging, with 50% of AI leaders implementing the technology in multiple key areas such as revenue generation, process automation, risk management, customer service, and client acquisition. All AI leaders expect to become mass adopters within two years, supporting the idea that significant economies of scale exist in applying AI to financial services.
Mass adoption seems to necessitate specialised organisational resources. Leading firms in AI implementation often establish dedicated departments to manage and deploy AI, while strategically involving a wider range of business functions.
"A century ago, factories electrified without rethinking their production lines and therefore saw no productivity benefits. In much the same way, machine learning technology without management and organisational change will be ineffective.” - Erik Brynjolfsson, Director of the MIT Initiative on the Digital Economy and Professor at MIT Sloan School of Management (Johnson, 2019)
Adoption challenges for Fintechs
Fintechs might face different challenges in integrating and scaling AI technologies across the organization, such as a lack of a clear AI strategy, an inflexible and investment-starved technology core, fragmented data assets, and outmoded operating models that impede collaboration between business and technology teams.
According to 2020' EY's survey, Fintechs prioritise the strategic role of AI in their businesses more than incumbents. However, both groups anticipate AI to be a major business driver within the next two years. Two years into the present, were they dam right!
Despite varying perceived strategic importance of generative AI impacting fintech across different financial service sectors, companies foresee AI becoming universally significant in the same timeframe, with the most significant growth expected in the payments sector.
Incumbents' expectations may be attributed to their shift from using AI for leaner, cost-effective operations to adopting differentiation strategies through process innovation and AI-powered customer service solutions.
In contrast, many Fintechs are already implementing differentiation-focused AI strategies to develop new products and services. A larger portion of Fintechs are also offering AI-powered products as a service, representing a unique AI-enabled business model that capitalizes on economies of scale in AI. This approach uses extensive and diverse data sets to provide AI-driven services through shared platforms (see some examples bellow).
As certain AI-powered solutions become commonplace, companies are motivated to utilize AI in creating truly innovative value propositions, establishing durable competitive advantages through product differentiation.
A larger proportion of Fintechs adopt a product-centric approach, selling AI-enabled services, while incumbents concentrate on using AI for process innovation within their existing product portfolios, some example business cases include the following:
Acornmachine — OakNorth's ACORN Machine utilizes alternative data sets to develop tailored loans for SMEs. In just two years, they have originated £800 million in loans and now offer their technology as a service to other lenders.
Ping An — Ping An's OneConnect is a comprehensive technology platform that leverages AI, blockchain, and big data analytics capabilities for various products, which are provided as a service to financial institutions of all sizes in China. To date, the platform has attracted a clientele that includes over 600 banks and 3,000 other financial institutions.
Neocova — Neocova offers a cloud-based core banking system for community banks and credit unions, incorporating AI in multiple applications such as anti-money laundering (AML).
BlackRock — BlackRock's Aladdin platform delivers 'Collective Intelligence' through a range of services for risk management, portfolio management, investment operations, and trade execution catered to diverse financial service providers. In 2019, the platform reportedly managed an aggregate of $17 trillion in assets (BlackRock, 2019).
AI implementation hurdles
AI relies on data to fuel its applications, allowing firms to scale their AI initiatives. However, access to and quality of data, as well as access to talent, remain significant challenges to AI implementation across industries, and Fintech is not exception.
Data quality issues may result in expensive processing steps or, in the worst-case scenario, render datasets unusable. Limited access to data might be due to organizations lacking the necessary infrastructure for data collection, storage, and transfer.
For AI Leaders, access to talent seems to be the most crucial obstacle, suggesting that advanced AI solutions require distinct employee competencies.
Investment managers face the most difficulty accessing data, likely due to their overall data-intensive usage profile. Payment providers typically express fewer concerns about barriers, which may be linked to the fact that many are not currently utilising AI as a central value proposition and might not be aware of potential impediments to AI implementation.
Although AI explainability issues are presently perceived as less obstructive than other barriers, these problems may become more evident as adoption increases and firms overcome initial hurdles to implementation.
With over a decade of hands-on expertise in strategic compliance guidance, iBerotech leverages regulatory technology and partnerships to empower foreign firms in skilfully navigating Spain's intricate regulatory environment for competitive advantage.
AI as a risk driver
Fintech executives believe that widespread AI adoption will introduce significant risks, particularly concerning data privacy and discrimination. At least a quarter of these firms do not feel well-prepared to address these risks.
Firms' evaluations of risks related to mass AI adoption are influenced by their views on AI as a consolidating or disruptive force. Those anticipating consolidation see AI generating industry-wide points of failure, while those expecting disruption focus on threats to market function and the pricing of assets.
A persistent gap exists between the anticipated market-level impact of AI adoption on risk and the effects that firms currently perceive. The latter are generally modest, with AI often acting as a net risk mitigator.
This perception gap cannot be simply attributed to ignorance or bias. It prevails regardless of firms' experience or resources, suggesting that companies likely foresee emerging risks under mass adoption that are not applicable today.
While regulation and the involvement of Risk and Compliance teams in AI implementation offer important assurances to firms, they may also create blind spots, leading firms to prioritise explicitly regulated risks over those that are not.
Although risk management is the most prevalent domain for AI application within firms, it remains unclear whether companies employing this approach have experienced better outcomes than their competitors.
Fintechs must to prioritise AI to stay ahead
For decades, banks have been pioneers in adapting new technology innovations to improve how they serve customers. From the introduction of ATMs in the 1960s to the adoption of mobile-based banking in the 2010s, banks have always stayed ahead of the curve. Today, we are in the AI-powered digital age, and Fintechs need to prioritise AI to stay ahead or sit back and inevitably die.
AI technologies have the potential to create $1 trillion in incremental value for Fintechs across several use cases. These technologies can help boost revenue, lower costs, and uncover new opportunities based on an unprecedented ability to process and generate insights from vast troves of data. Fintechs that fail to become “AI-first” risk being left behind by their customers and overtaken by competition, inevitably.
Disruptive AI technologies can dramatically improve Fintechs’ ability to achieve four key outcomes: higher profits (initially by by cutting costs), at-scale personalisation, distinctive omnichannel experiences, and rapid innovation cycles.
Four current trends are accentuating the need for Fintechs to prioritise AI. First, rising customer expectations as adoption of digital banking services increases. Second, leading financial institutions’ use of advanced AI technologies is steadily increasing with 80% of surveyed firms already implementing some degree of AI back in 2020. Third, digital ecosystems are disintermediating traditional financial services. Finally, technology giants are entering financial services as the next adjacency to their core business models.
Fintechs must embrace AI to stay competitive and meet the growing expectations of their customers. By prioritising AI, Fintechs can unlock the potential for value creation and stay ahead of the curve.
Deploying AI solutions
As Fintechs strive to keep pace with rising customer expectations and fend off competitors in the digital age, the AI-first Fintech of the future will provide intelligent propositions and experiences that are personalised and truly omnichannel, seamlessly blending banking capabilities with non-banking products and services. Through advanced diagnostic engines and the broad application of traditional and leading-edge AI technologies like machine learning and facial recognition, the AI-first Fintech will achieve operational efficiency and enhance customer experience in ways that is hard to imagine.
Internally, the AI-first Fintech will be optimised for operational efficiency, employing a "zero-ops" mindset to automate manual tasks and replace human decision-making with advanced diagnostic engines across diverse areas of Fintech operations. This approach will enable the Fintech to analyse large and complex reserves of customer data in (near) real time, resulting in significant gains in operational performance, directly affecting the bottom line.
The AI-first Fintech will also enjoy the speed and agility that is the hallmark of digital-native companies, innovating rapidly and launching new features in a matter of days or weeks instead of months. Through extensive collaboration with partners, the AI-first Fintech will seamlessly integrate new value propositions across journeys, technology platforms, and data sets.
As Fintech executives, we must overcome a key challenge in order to deploy AI capabilities at scale. We must balance the need for speed, agility, and flexibility inherent to fintechs with the continued management of scale, security standards, and regulatory requirements of a traditional financial-services enterprise.
"In Fintech, one of the main obstacles hampering development efforts is the lack of a clear strategy for Generative AI." — Julio Gomez
Often, Fintechs' core technology systems have performed well in supporting traditional payments and lending operations. However, disruptors must resolve several inherent weaknesses before we can deploy AI technologies at scale. These systems often lack the capacity and flexibility required to support the variable computing requirements, data-processing needs, and real-time analysis that closed-loop AI applications require.
In addition, core systems are difficult to change, and their maintenance requires significant resources. More often than not, data reserves are fragmented across multiple silos, and analytics efforts are focused narrowly on stand-alone use cases. Without a centralised data backbone, it is practically impossible to analyse the relevant data and generate an intelligent recommendation or offer at the right moment, and place.
Executives must govern and make data available securely in a manner that enables analysis of data from internal and external sources at scale for millions of customers, in (near) real-time, at the “point of decision” across the organisation.
Lastly, these winning organisations must have a robust set of tools and standardised processes to build, test, deploy, and monitor models in a repeatable and “industrial” way.
Traditional operating models further impede efforts to meet the need for continuous innovation. Organizations are often organised around distinct business lines, with centralised technology and analytics teams structured as cost centers. Business owners define goals unilaterally, and alignment with the enterprise’s technology and analytics strategy (where it exists) is often weak or inadequate.
Siloed working teams and “waterfall” implementation processes invariably lead to delays, cost overruns, and suboptimal performance. Additionally, they lack a test-and-learn mindset and robust feedback loops that promote rapid experimentation and iterative improvement. Executives must prioritise the development of in-house capabilities and talent to ensure competitive differentiation and avoid reliance on third-party technology providers for critical functionalities.
Actionable recommendations
As Fintechs seek to keep pace with the rapid advances in AI technology, they must adopt a holistic approach to transformation, investing in what McKinsey describers as "the four layers of the integrated capability stack". To become AI-first, Fintechs must reimagine their customer engagement layer, the AI-powered decision-making layer, the core technology and data layer, and the operating model.
The first layer involves reimagining customer engagement, providing personalised solutions that go beyond traditional financial services products. This means creating integrated propositions that target "jobs to be done," embedding personalization decisions within core customer journeys, and integrating non-banking products and services to comprehensively address customer needs. To achieve this, Fintechs must adopt a design-thinking approach, engineering engagement interfaces for flexibility and granularly embedding data-capture funnels to enable continuous improvement.
The second layer involves building an AI-powered decision-making layer, using AI techniques to automate decisions and produce better outcomes. Fintechs must establish an enterprise-wide road map for deploying advanced-analytics/machine-learning models, making the development process repeatable, and rewiring business processes to embed AI decisioning. In addition, Fintechs should integrate emerging capabilities, such as natural-language processing and computer-vision techniques, from specialist providers at rapid speed through an architecture enabled by an API.
To deliver these decisions and capabilities and engage customers across the full life cycle, Fintechs must establish enterprise-wide digital marketing machinery. This machinery requires data-ingestion pipelines, data platforms that maintain a 360-degree view of customers and enable AA/ML models to run and execute in near real time, and campaign platforms that coordinate interventions across the range of channels in the engagement layer.
The fourth layer involves transitioning to the platform operating model, breaking up organisational silos and increasing agility and speed by integrating business and technology in jointly owned platforms run by cross-functional teams. This requires Fintechs to establish three archetypes of platform teams: business platforms, enterprise platforms, and enabling platforms.
As Fintechs embrace AI, becoming an AI-first organisation requires a comprehensive transformation across all layers of the capability stack. Neglecting or underinvesting in any layer can have a domino effect throughout the organisation, leading to a suboptimal stack that fails to meet enterprise objectives.
To start this journey, Fintech leaders should consider how AI technologies can help achieve their strategic goals, such as driving growth, enhancing profitability, improving customer engagement, and fostering innovation.
They should then align their AI goals with the strategic goals of the organisation. Following this, they should perform a comprehensive diagnostic to evaluate the current state of the organization across the four layers and identify areas that require key shifts, additional investments, and new talent. They can then leverage these insights to develop a transformation roadmap that encompasses business, technology, and analytics teams.
To ensure the transformation is sustainable, Fintechs should adopt a two-track approach that balances short-term projects that deliver business value every quarter with a continuous build of long-term institutional capabilities.
Depending on their market position, size, and aspirations, Fintechs may not need to build all capabilities themselves. They can choose to retain differentiating core capabilities in-house while acquiring non-differentiating capabilities from technology vendors and partners, including AI experts.
In conclusion, to become AI-first, Fintechs must adopt a holistic approach, investing in all four layers of the integrated capability stack. By doing so, Fintechs can reimagine customer engagement, build an AI-powered decision-making layer, establish enterprise-wide digital marketing machinery, and transition to the platform operating model, increasing agility and speed, and improving the alignment of goals and priorities across the enterprise.
For Fintechs, adopting AI technologies across the enterprise is no longer optional, but rather a strategic imperative. Success in this endeavor requires a holistic approach that considers all four layers of the capability stack.
Over the years, we have gained a reputation on strategic compliance guidance.
At iBerotech, we harness regulatory expertise and leverage key strategic partnerships to empower foreign financial services companies in adroitly traversing Spain’s multifaceted regulatory landscape for strategic growth.